How we use predictive analytics to improve our clients' experience
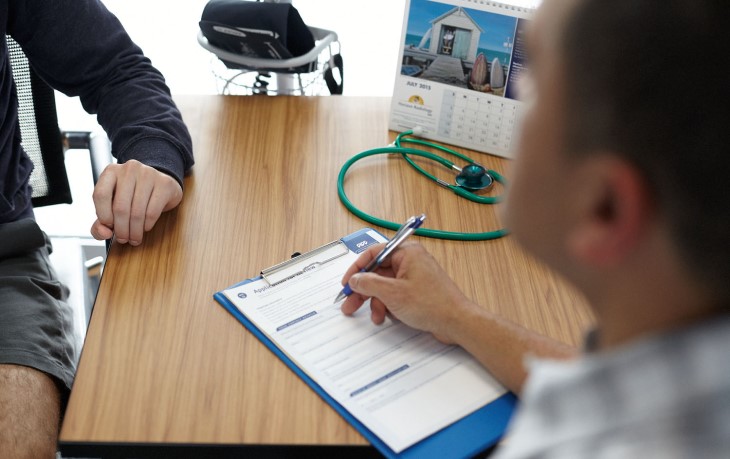
Our predictive modelling tool helps us identify clients who need our assistance over and above paying for treatment.
ACC's use of a predictive analytics tool has been in the news recently, with some coverage suggesting we use analytics to 'target' clients, and send them back to work before they had recovered.
That is definitely not the way we do things here, but we acknowledge we should be doing a better job at explaining how we use the data we collect from claims information to improve our clients' experience and outcomes.
We have a number of tools to help us help our clients in the best way possible, and one of the most important tools is data and analytics. We get approximately 2 million claims each year and the information collected on these tells us a lot about how people are injured, how long it takes them to recover and what kind of help is needed for them to get back on with their lives.
A predictive modelling tool based on that data helps us to rapidly identify the claims where clients are likely to need our assistance over and above paying for treatment. Engaging quickly with these clients is important, as it can be an uncertain time for them following an accident, and previously some of them had to ring us if they needed help.
Modelling can predict the time it will take someone to recover from injury and be fit to return to work. This helps case owners provide clients with initial expectations, although ultimately a client's recovery timeframe is determined by medical providers.
Since we started using our predictive analytics tool, client satisfaction has gone from 68% in June 2013 to 78%, and the average time taken to set up weekly compensation payments has dropped from 11 days in June 2014 to seven days now.
The tool is not a key performance indicator for staff. Case owners do not have individual duration targets but work towards local and regional-level targets in order to optimise outcomes for clients.
Individual clients are not able to be identified in the tool, and our injury claim forms' privacy notice outlines our role and responsibility in collecting data and personal information.
More about our privacy notice and how we protect your privacy
The data collected is for claims lodged after April 2009 and is verified via statistical tests. Factors used in the model include:
- diagnosis
- age
- lodgment delay
- work type (if relevant)
- questions about whether treatment was sought at a DHB
- a client's injury history (eg whether they've had multiple injuries on the same body site)
- initial incapacity outlined on the claim form.
We do not use ethnicity or gender as factors.
The model was reviewed externally in 2013, and calibration assessments to confirm its accuracy were done in 2016 and 2017. No other agencies have access to the data.
Read the external review of the model
In 2015 global technology company FINEOS awarded ACC's predictive modelling tool its Innovation and Excellence Award.
If anyone has any concern about any matter arising out of this work, they're always very welcome to contact us.